Insulator Defect Detection based on Faster R-CNN and YOLOv3 Algorithm
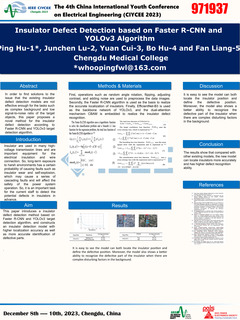
Start Time:Pending (Asia/Shanghai)
Duration:Pending
Session:[No Session] » [No Session Block]
Tips: The file permissions under this presentation are only for participants. You have not logged in yet and cannot view it temporarily.
Abstract
Keywords
Speaker

Comment submit