Time Series Prediction of day-ahead Photovoltaic Power Based on Data-Driven
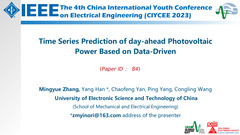
Start Time:2023-12-09 09:45 (Asia/Shanghai)
Duration:15min
Session:[S1] Renewable energy system » [S1] Renewable energy system
Tips: The file permissions under this presentation are only for participants. You have not logged in yet and cannot view it temporarily.
Abstract
Keywords
Speaker

Comment submit